AI, zBlog
Generative AI vs Traditional AI: Contrasting Innovation and Legacy
trantorindia | Updated: April 24, 2024
Introduction
In the evolving landscape of artificial intelligence (AI), the dichotomy between generative AI vs traditional AI delineates a remarkable transformation. The field of artificial intelligence (AI) has undergone a remarkable transformation in recent years, with the emergence of generative AI marking a significant departure from traditional AI approaches. While traditional AI systems have been instrumental in tackling a wide range of tasks, generative AI promises to push the boundaries of what is possible, unlocking new realms of creativity, efficiency, and innovation.
In this comprehensive article, we will delve into the contrasts between generative AI vs traditional AI, exploring their respective strengths, limitations, and potential implications for various industries and disciplines. By understanding the nuances of these two AI paradigms, we can better appreciate the transformative power of generative AI and its capacity to reshape the way we approach problem-solving, decision-making, and the creation of content and artifacts.
Traditional AI: The Foundations of Machine Intelligence
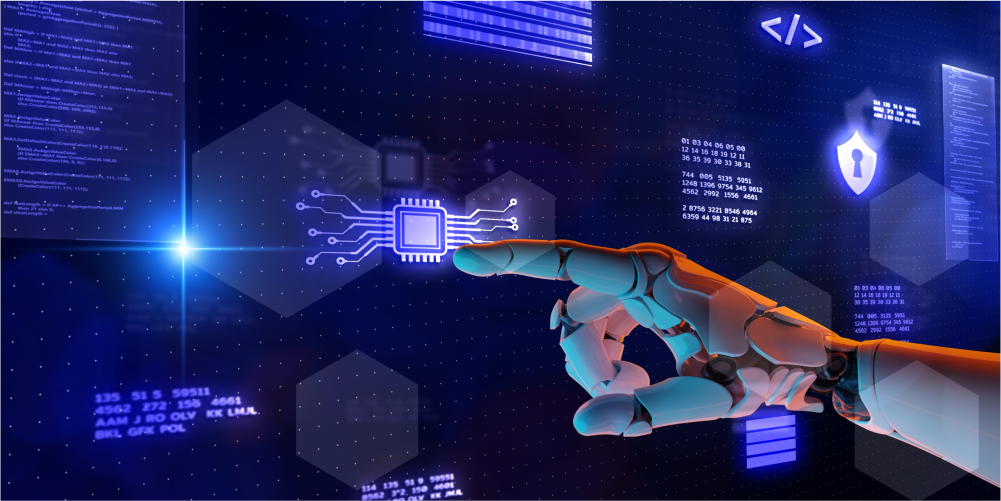
Traditional AI, also known as discriminative or analytical AI, has been the dominant paradigm in the field for decades. These systems are designed to perform specific tasks, such as classification, prediction, and decision-making, based on predefined rules and algorithms. Traditional AI models are trained on large datasets, learning to recognize patterns and make decisions based on the input data.
The core principles of traditional AI include:
- 1. Rule-based systems: Traditional AI systems often rely on predefined rules and heuristics, which are manually coded by human experts. These rules are designed to mimic human decision-making processes and are used to solve specific problems or tasks.
- 2. Machine learning: Traditional AI heavily leverages machine learning techniques, such as supervised learning, unsupervised learning, and reinforcement learning. These algorithms are trained on labeled or unlabeled data to identify patterns and make predictions or decisions based on the input data.
- 3. Expert systems: Expert systems are a type of traditional AI that emulates the decision-making ability of human experts in a particular domain. These systems leverage knowledge bases and inference engines to solve complex problems and provide recommendations or solutions.
- 4. Natural Language Processing (NLP): Traditional AI has made significant strides in the field of NLP, enabling systems to understand, interpret, and generate human language. This has led to the development of applications such as language translation, sentiment analysis, and conversational agents (chatbots).
- 5. Computer vision: Another area where traditional AI has excelled is computer vision, which involves the analysis and interpretation of visual data, such as images and videos. Applications include object recognition, facial recognition, and autonomous vehicle navigation.
While traditional AI has achieved remarkable success in many domains, it is primarily focused on solving specific, well-defined problems and tasks. These systems excel at processing structured data and making decisions based on predefined rules and patterns, but they often struggle with open-ended, creative tasks and lack the ability to truly understand and reason about the world in the way that humans do.
Generative AI: Unlocking Creativity and Innovation
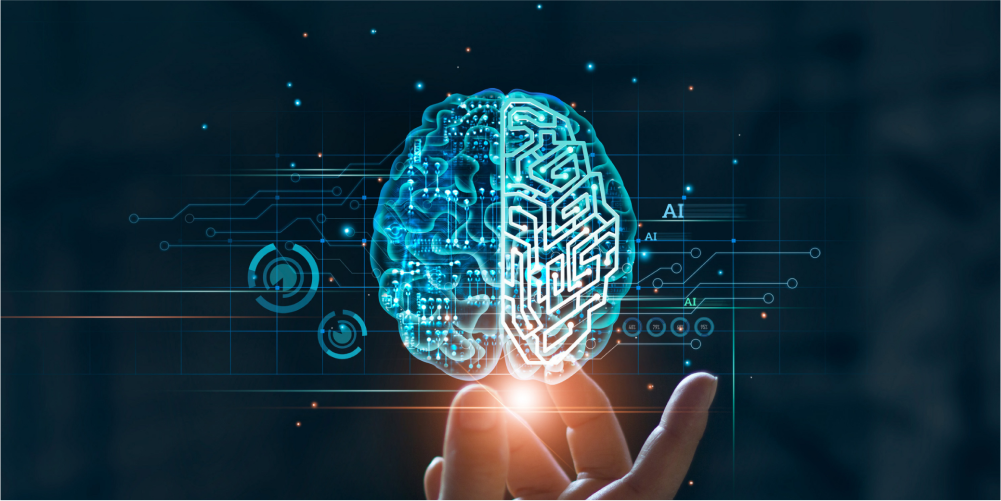
Generative AI, also known as creative AI or generative models, represents a paradigm shift in the field of artificial intelligence. Unlike traditional AI systems that are designed to perform specific tasks, generative AI models are trained to create new content or artifacts from scratch, based on the patterns and relationships they have learned from the training data.
The core principles of generative AI include:
- 1. Unsupervised learning: Generative AI models are often trained using unsupervised learning techniques, which allow them to discover patterns and relationships in the data without relying on labeled examples or predefined rules.
- 2. Generative models: Generative models, such as Generative Adversarial Networks (GANs), Variational Autoencoders (VAEs), and Diffusion Models, are at the heart of generative AI. These models learn to generate new data samples that resemble the training data, enabling the creation of novel content or artifacts.
- 3. Latent space exploration: Generative AI models can navigate and explore the latent space, which represents the underlying structure and relationships within the data. By manipulating the latent space, these models can generate diverse and novel outputs while preserving the essential characteristics of the training data.
- 4. Multimodal learning: Generative AI models can learn from and generate content across multiple modalities, such as text, images, audio, and video. This enables the creation of rich, multisensory experiences and opens up new possibilities for creative expression.
- 5. Open-ended exploration: Unlike traditional AI systems that are constrained by predefined rules and objectives, generative AI models can explore open-ended tasks and domains, fostering creativity and enabling the discovery of novel solutions and ideas.
The applications of generative AI are vast and far-reaching, spanning various industries and disciplines. Some notable examples include:
- 1. Content creation: Generative AI models can be used to create original text, images, music, and even video content, revolutionizing the creative industries and enabling new forms of artistic expression.
- 2. Scientific discovery: By exploring the latent space and generating novel data samples, generative AI models can aid in the discovery of new materials, drug candidates, and scientific insights, accelerating research and development processes.
- 3. Product design and innovation: Generative AI can be leveraged to design and optimize products, from consumer goods to architectural structures, by exploring and generating novel designs that meet specific criteria and constraints.
- 4. Simulation and virtual environments: Generative models can be used to create realistic virtual environments and simulations, enabling training and testing in various domains, such as autonomous vehicles, robotics, and gaming.
- 5. Data augmentation: Generative AI can be used to augment existing datasets, creating synthetic data samples that can be used to train and improve the performance of traditional AI models, particularly in data-scarce domains.
While generative AI holds immense potential, it also presents several challenges and ethical considerations that must be addressed. These include the risk of generating biased or harmful content, the potential for misuse (e.g., deepfakes), and the need for robust evaluation and quality control mechanisms.
Contrasting Generative AI vs Traditional AI
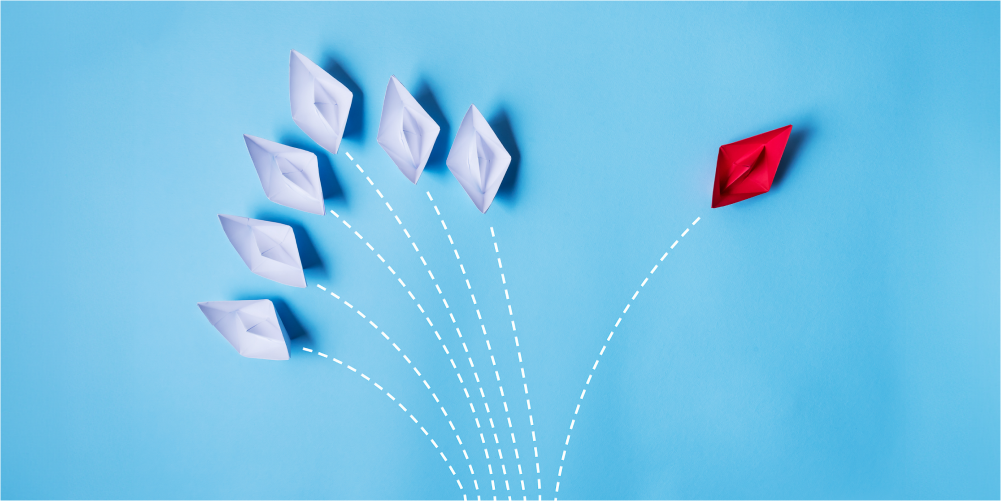
While both generative AI and traditional AI share the common goal of enabling intelligent systems, they differ significantly in their approaches, capabilities, and potential impact. Here are some key contrasts between the two paradigms:
- 1. Problem-solving approach: Traditional AI is primarily focused on solving specific, well-defined problems through rule-based systems and machine learning algorithms. Generative AI, on the other hand, takes a more open-ended and exploratory approach, enabling the creation of novel content and artifacts.
- 2. Creativity and innovation: Generative AI models are designed to foster creativity and innovation by exploring the latent space and generating novel outputs. Traditional AI systems, while powerful, are typically constrained by predefined rules and objectives, limiting their ability to generate truly novel and creative solutions.
- 3. Data requirements: Traditional AI models often require large, labeled datasets for training, which can be time-consuming and resource-intensive to acquire. Generative AI models, on the other hand, can leverage unsupervised learning techniques and learn from unlabeled or partially labeled data, potentially reducing the data requirements.
- 4. Interpretability and transparency: Traditional AI models, particularly those based on rule-based systems and interpretable machine learning algorithms, can offer greater transparency and interpretability, making it easier to understand their decision-making processes. Generative AI models, however, can be more opaque, with their outputs emerging from complex interactions within the latent space.
- 5. Ethical considerations: Both traditional AI and generative AI present ethical challenges, but generative AI introduces unique concerns related to the potential for generating biased, harmful, or deceptive content, as well as issues around intellectual property and copyright.
- 6. Scalability and efficiency: Generative AI models can potentially be more scalable and efficient than traditional AI systems in certain domains. For example, in content creation, a single generative model could generate vast amounts of diverse content, outpacing traditional methods.
- 7. Domain adaptability: Traditional AI systems are often designed and optimized for specific domains or tasks, making it challenging to adapt them to new domains or problem spaces. Generative AI models, with their ability to learn and generate from patterns in the data, may be more adaptable to new domains and tasks, albeit with potential challenges in maintaining quality and consistency.
The interplay between generative AI vs traditional AI is likely to shape the future of artificial intelligence. While traditional AI will continue to play a crucial role in solving well-defined problems and tasks, generative AI has the potential to unlock new realms of creativity, innovation, and discovery. By combining the strengths of both paradigms and addressing their respective limitations, we can pave the way for more powerful and transformative AI systems that enhance human capabilities and drive progress across various domains.
Conclusion
At Trantor, we recognize the pivotal role of both generative AI vs traditional AI in shaping the future of technology. We are at the forefront of leveraging cutting-edge technologies like generative AI, machine learning, automation, and robotic process automation (RPA) to drive innovation and digital transformation for our clients. Our comprehensive suite of AI-powered solutions harness the power of generative models, advanced analytics, and intelligent automation to unlock new efficiencies, enhance decision-making, and enable novel applications across industries. With our deep expertise in Artificial Intelligence and Machine Learning research and product engineering, combined with our robust automation capabilities, Trantor is uniquely positioned to help organizations navigate the challenges of today while preparing them for the opportunities of tomorrow through the responsible adoption of disruptive technologies.
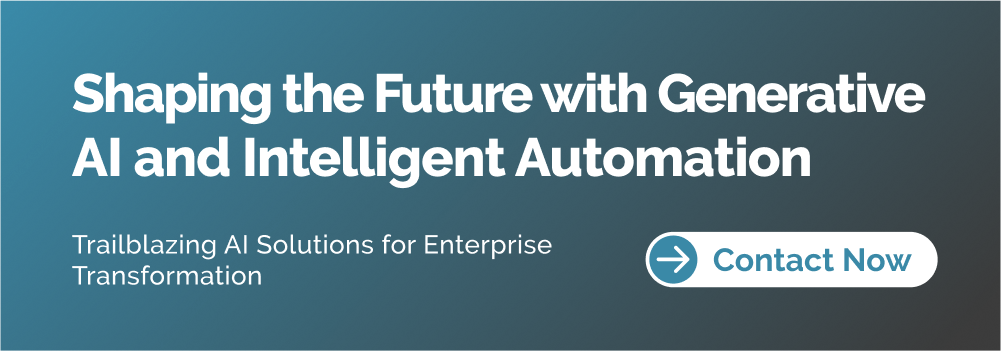